Highlights
- Choose your RAG architecture based on query complexity—VectorRAG for semantic similarity, GraphRAG for relationship-intensive domains requiring multi-hop reasoning with explicit entity connections.
- Implement granular performance metrics (retrieval precision, recall@k, latency distributions) to quantify the actual benefits of each approach for your specific workloads.
- Consider hybrid architectures that leverage VectorRAG for broad retrieval and GraphRAG for relationship verification, accepting the 150-200ms orchestration overhead for 15-25% accuracy gains.
Enterprise AI teams building Retrieval-Augmented Generation (RAG) systems must choose between VectorRAG and GraphRAG architectures. Each approach presents distinct technical challenges that affect accuracy, scalability, and query complexity.
- VectorRAG relies on embedding-based similarity, offering efficient broad retrieval but struggling with structured multi-hop reasoning.
- GraphRAG explicitly encodes entity relationships, improving logical consistency but introducing higher upfront schema complexity.
This article analyzes the core engineering challenges of both approaches, covering multi-hop reasoning, update mechanisms, schema trade-offs, query performance, and explainability.
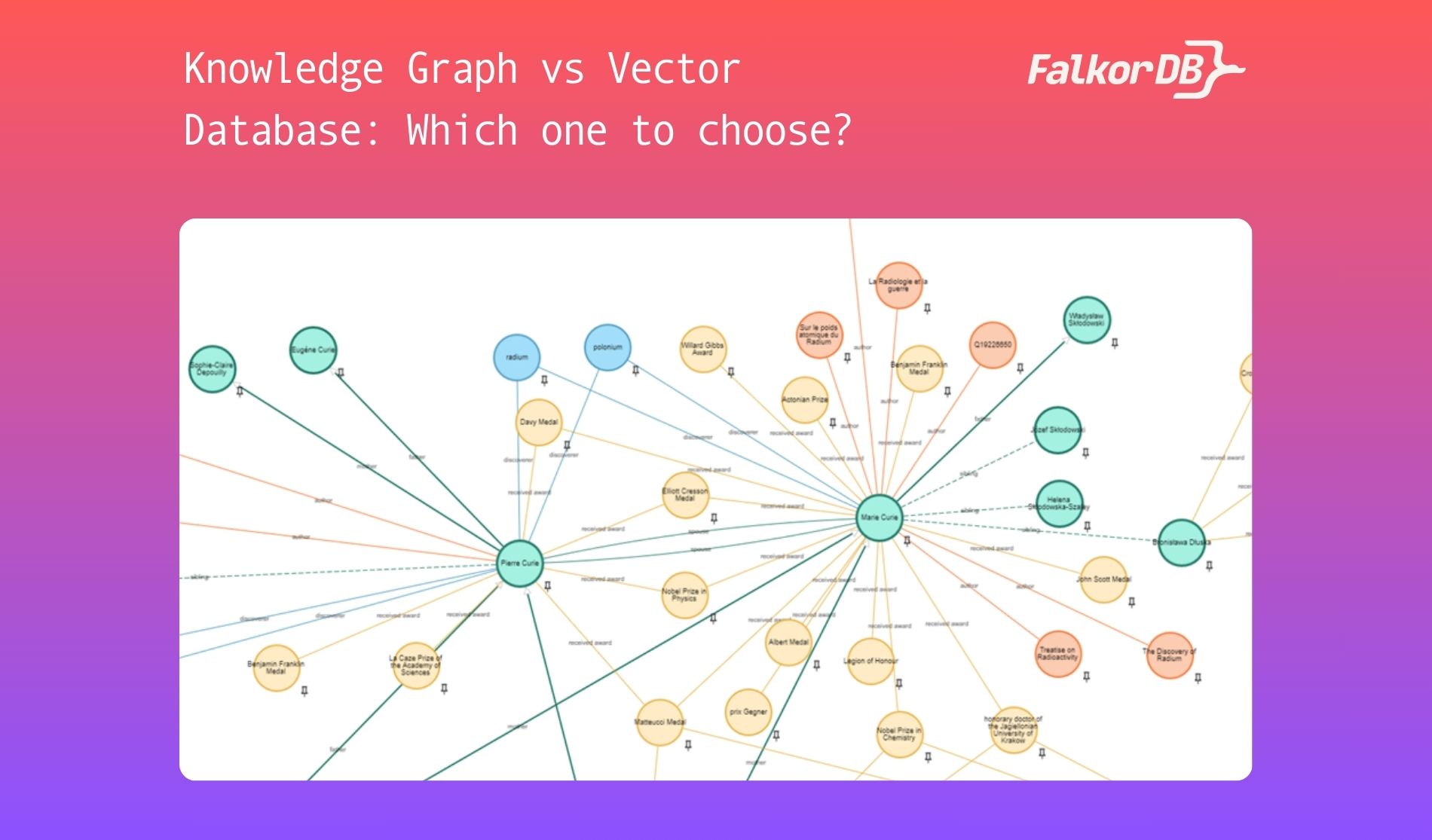
Multi-Hop Reasoning: Structural Constraints and Scalability
VectorRAG Limitations
Vector-based retrieval operates on semantic similarity, which inherently flattens hierarchical information. This makes multi-step reasoning computationally expensive and prone to accuracy degradation.
- Embeddings lack explicit structural awareness, forcing reliance on approximate similarity.
- Queries requiring deep logical connections—e.g., tracing ESG controversies affecting a supplier’s financials—suffer from connection loss in high-dimensional space.
- Performance degrades exponentially beyond two to three logical hops, making deep reasoning unreliable.
GraphRAG Strengths and Complexity
Graph-based retrieval enables direct traversal of structured relationships (e.g., Company → Supplier → ESG Violation → Financial Impact
).
- Path-based reasoning preserves logical connections, ensuring accuracy across deep multi-hop queries.
- Schema complexity grows with depth—more relationships require precise ontological design.
- Implementation requires significant upfront effort in defining relationship types, constraints, and traversal rules.
Hybrid Approaches
Some architectures use vector similarity for candidate selection, followed by graph verification to confirm structured relationships. This balances broad recall with logical precision.
Data Updates: Real-Time Constraints and Computational Overhead
VectorRAG: Re-Embedding Challenges
Updating vectorized data requires full re-embedding, leading to O(n) computational complexity.
- Partial updates risk embedding drift, where inconsistencies arise between newly added and pre-existing vectors.
- Continuous data streams (e.g., financial news, legal filings) face high reprocessing costs.
- Embedding recalibration often requires offline batch processing, delaying real-time updates.
GraphRAG: Granular Incremental Updates
Graph-based architectures support node- and edge-level updates, avoiding full reprocessing.
- Temporal edge versioning allows tracking of relationship evolution (e.g., contract amendments).
- Graph synchronization requires custom consistency mechanisms, especially in distributed environments.
Optimization Strategies
- VectorRAG: Batch reprocessing with change-detection algorithms minimizes unnecessary updates.
- GraphRAG: Temporal partitioning and incremental graph expansion optimize update efficiency.
Schema Flexibility vs. Query Precision
VectorRAG: Schema-Agnostic Flexibility
Vector-based systems simplify ingestion but risk semantic dilution—unrelated concepts with similar embeddings may cluster incorrectly.
- Example: “Java” (island) vs. “Java” (programming language) in a technical search.
- Disambiguation requires embedding tuning, increasing implementation complexity.
GraphRAG: Schema-Dependent Rigor
Graph models enforce strict entity-relationship rules, improving precision but requiring ongoing ontology alignment.
- Example: Pharmaceutical R&D models must manually curate Compound → Target Protein → Pathway relationships.
- Schema evolution becomes a long-term maintenance challenge.
Domain-Specific Schema Considerations
- Finance: Regulatory compliance and risk modeling require explicit entity relationships.
- Healthcare: Standardized ontologies (SNOMED CT, RxNorm) ensure precise data linkage.
- Manufacturing: Temporal tracking of supply chain relationships demands structured updates.
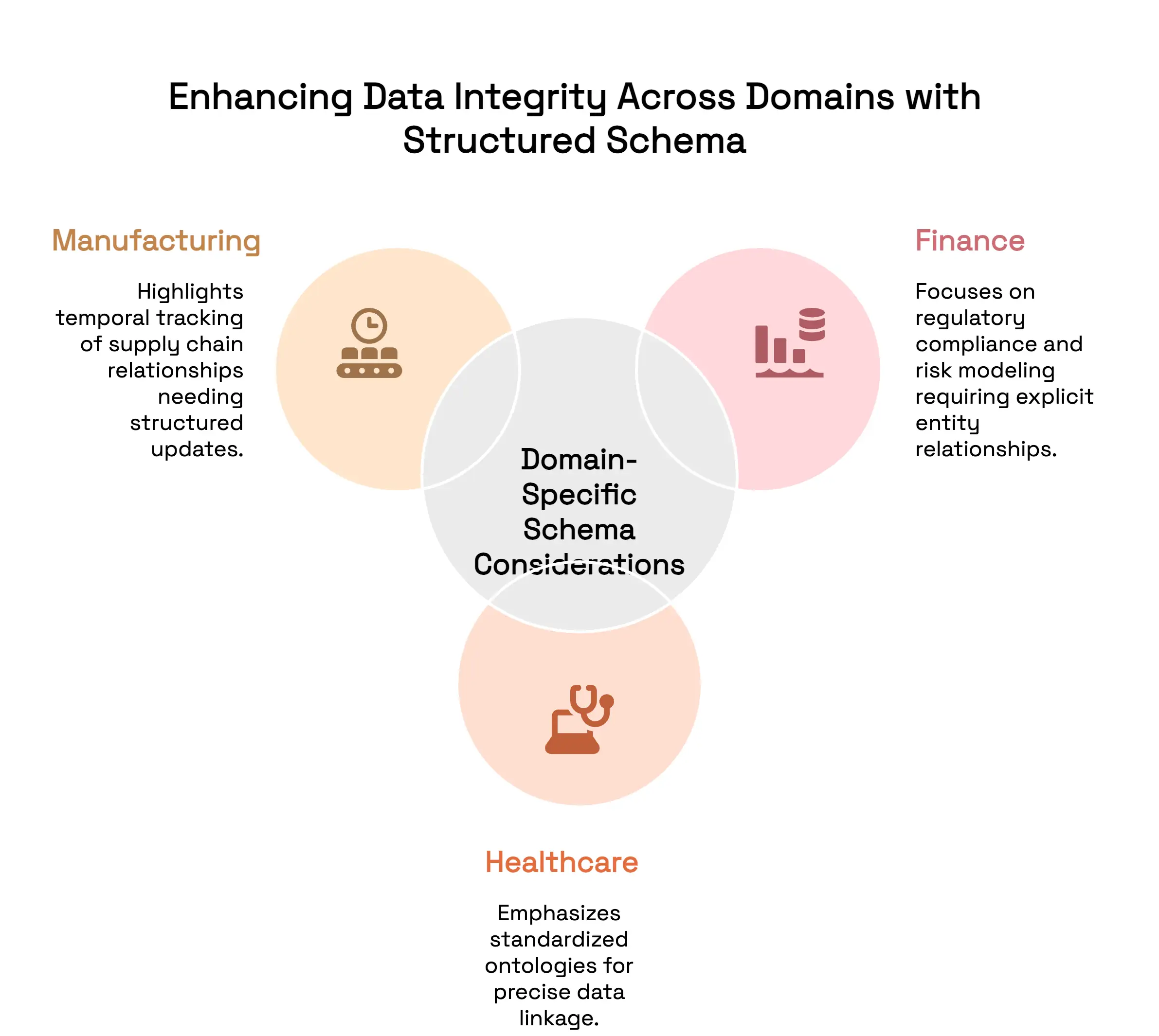
Query Performance and Latency Optimization
Vector Search: Speed vs. Recall Trade-offs
Vector-based retrieval achieves sub-second latency using Approximate Nearest Neighbor (ANN) algorithms (e.g., HNSW).
- Speed comes at the cost of recall precision—important legal or compliance queries risk missing critical documents.
- Sliding window chunking helps mitigate noise but fragments logical dependencies.
Graph Query Optimization
Graph queries use index-assisted traversal, reducing latency but struggling with dense subgraph bottlenecks.
- Multi-hop queries (e.g., tracking supply chain dependencies) can exceed 300ms, limiting real-time feasibility.
- Query planning and cache-based acceleration improve response times.
Hardware Acceleration
- VectorRAG: GPU acceleration speeds up similarity calculations.
- GraphRAG: Specialized graph processors enhance complex traversals.
- Memory-optimized architectures support billion-node graphs efficiently.
Entity Disambiguation at Scale
VectorRAG: Embedding Disambiguation Challenges
Semantic embeddings struggle with polysemy—words with multiple meanings.
- Example: “Apple” (fruit) vs. “Apple” (company).
- Sense-specific embeddings require manual data tagging, increasing complexity.
GraphRAG: Context-Aware Disambiguation
Graphs resolve entities by leveraging surrounding context.
- Example: A node labeled “Apple” connected to Cupertino and iPhone resolves ambiguity.
- Indexing large-scale graphs significantly increases memory requirements.
Structural Information Preservation
VectorRAG: Information Loss
Vectors flatten syntactic structure, discarding critical elements like negation (“not compliant”).
- Fine-tuning positional encoding partially restores structure but increases computational overhead.
GraphRAG: Explicit Structural Integrity
Graphs maintain structural integrity through direct relationships (e.g., Regulation → Compliance Check).
- NLP-based graph extraction has 15-20% error rates, requiring post-processing verification.
Explainability and Compliance
VectorRAG: Opaque Similarity Scores
Vector retrieval lacks transparent logic, complicating compliance audits.
- Post-hoc explanation models (e.g., LIME) introduce latency and only approximate retrieval reasoning.
GraphRAG: Native Explainability
Graph retrieval produces explicit query paths (e.g., Patient → Prescription → Drug Interaction).
- Path explosion in cyclic graphs (corporate ownership loops) increases complexity.
- Query visualization is necessary for human interpretability.
Key Takeaways
- Industry-Specific Trade-offs: GraphRAG is better suited for relationship-intensive domains (finance, healthcare, supply chain), while VectorRAG works well for content-heavy applications.
- Measurement-Driven Implementation: Teams should rely on retrieval precision, recall@k, and latency metrics rather than theoretical advantages.
- Modular Design: The most effective systems combine vector search for recall with graph-based reasoning for logical consistency.